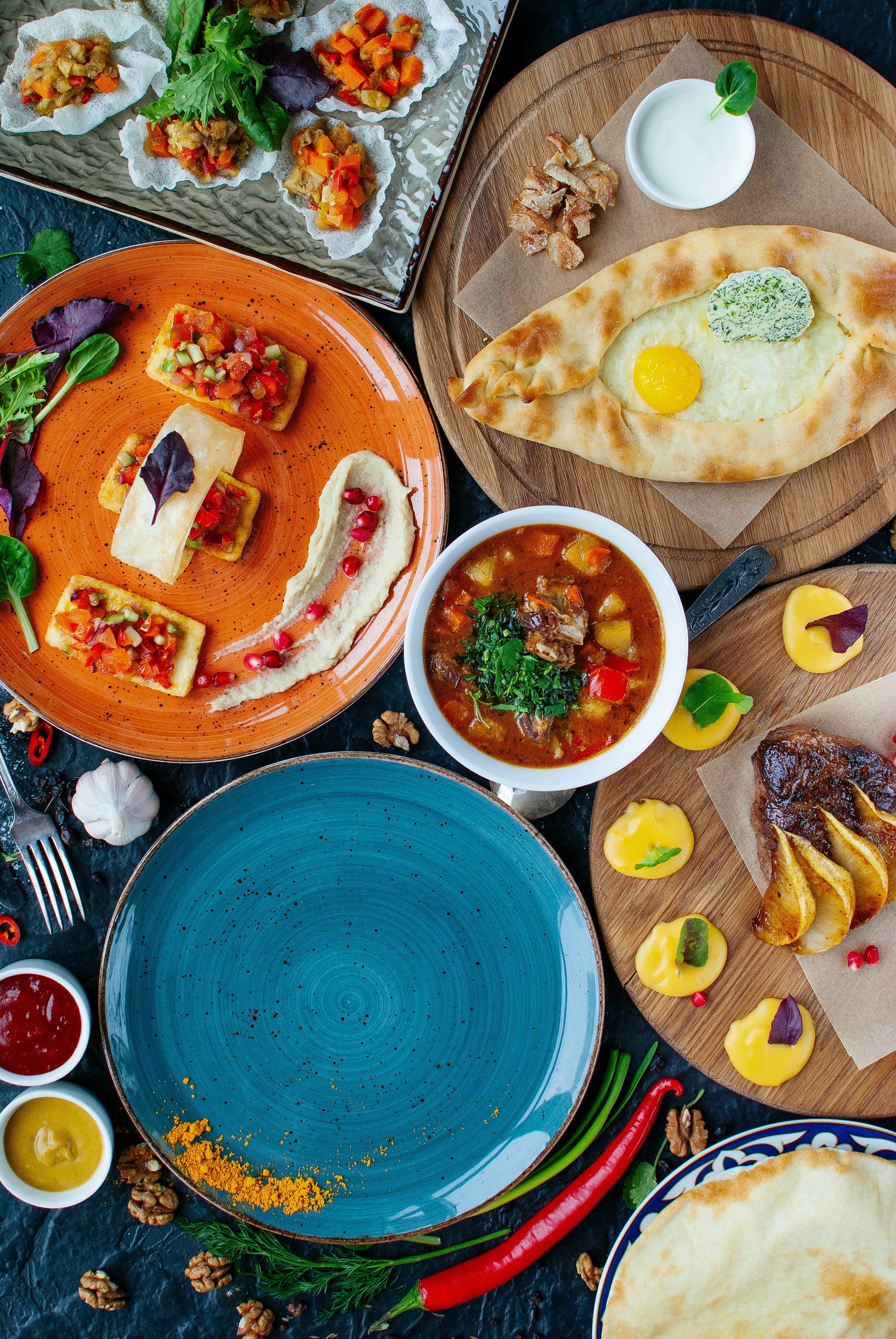
Vision - Food Image Classifier.
For this project I built a food classifier to identify food from different food images. This project aim was to beat 77.4% top-1 accuracy of DeepFood paper. I was able to get the model to predict the class of the food from 101 classes with 70% accuracy with out fine-tuning and 80% with fine-tuned model.
To get these results I used transfer learning (no fine-tuning) on a existing noncomplex pretrained (on ImageNet) Convolutional Neural Network (CNN) model "EfficienetNetB0". This created time efficiencies and solid results. Further experiments are needed to improve the accuracy like using more complex pretrained models (EfficienetNetB4 or ResNet34).
More Details
I started this project with binary classification problem by making a model that was able to classify Pizza or steaks foods. This experiment was also done on a simple CNN models, having no more then 2 Conv2D (8 - 16 filters) hidden layers and AveragePooling layer. These models were able to achieve above 85% accuracy. After simple experiments I started adding more classes and did two main experiments, first with 10 classes and last with 101 classes. The target goal was to beat two papers, 50.76% top-1 accuracy of "Food101 paper" and 77.4% top-1 accuracy of "Deepfood paper" that were also build on the same datasets. Details are in the following sections.
Analysis and Prediction
To understand results and the decision function I also build Confusion Matrix between 101 classes and also calculated F1-score of each class. To view these graphs and top most wrong predictions please visit my GitHub page, as these graphs were of high dimensions so I didn't uploaded them on site.
Also other then test data I used custom images which were collected from different. These images were also preprocessed to match there dimension, shape and scale. Out of 10 model predicted all correct with high probability scores.
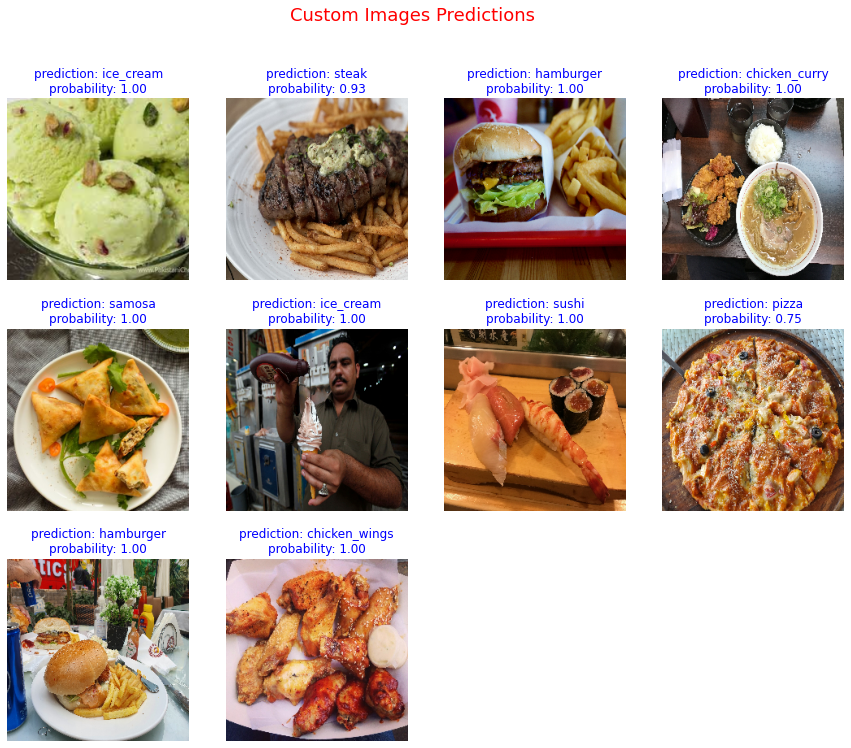
To view the source code, evaluation metrics (Precision, Recall, F1-score), Confusion Matrix and Top wrong predictions, please visit my Github page.